Groendus: Building AI driven energy forecasting capabilities
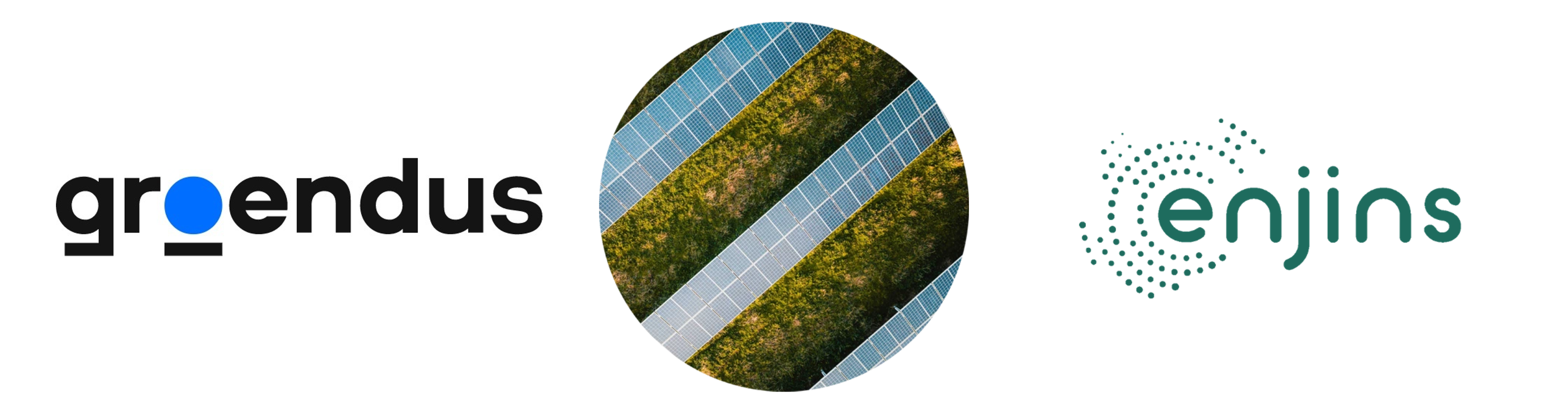
Realizing the energy forecasting capability of Groendus: 4 key lessons learned
Introduction: Groendus, being the one-stop-B2B-shop for energy needs, aims to optimize affordable and green energy for their clients. Since the rise of renewables and the continued decentralization and digitalization of grids, the volatility of the market increases, which makes it more difficult for clients to stay in control of their energy needs.
As a solution, Groendus offers their clients to gain insights into their energy usage: everything starts with trustworthy data. This data is translated into practical advice to reduce energy costs. Moreover, through Groendus Energiesturing that is equipped with machine learning algorithms, clients can steer their energy consumption and generation to increase the percentage of renewable energy used and reduce costs.
Underlying these services and tools, Groendus identified that data and artificial intelligence (AI) is a core capability. The better one can predict the future, the better their clients can proactively steer their asset within a highly volatile grid. And as a result, maximize green energy usage at an affordable price.
However, the challenge when building AI solutions within the energy sector is that the number of machine learning algorithms explode quite rapidly, from energy consumption to energy production, from volume to price predictions, from solar panels to other assets like batteries, and from long-term forecasts to short-term forecasts in PTU’s (15 minute Programme Time Unit). Hence, the question is, how to build an AI based energy forecasting system that can accommodate all these different algorithms whilst staying in control and iterating fast. And second, where to start?
End of Q4 2022, Groendus and Enjins sat down together to kick-off their data & AI strategy. Over the course of less than a year, the team co-developed a robust data analytics platform, including a ML ops platform. This enables Groendus to develop both analytics and machine learning use cases swiftly. Moreover, the joint team built the most important algorithms successfully, among others the energy consumption and production forecasts. Lastly, the capability to build new ML use cases and iterate on existing ones, now fully lies within the Groendus team.
This article shares our 4 key lessons learned
(1) While building an energy scale-up, make solid decisions on what you buy versus what you build in house.
(2) Utilizing internal data results in outperforming Groendus’ existing standard for energy consumption forecasting.
(3) Spend as much time on a ML ops platform as spending time on building your first algorithm.
(4) To scale Groendus’ analytics capability, analytics engineering (and analytics engineers) is key.
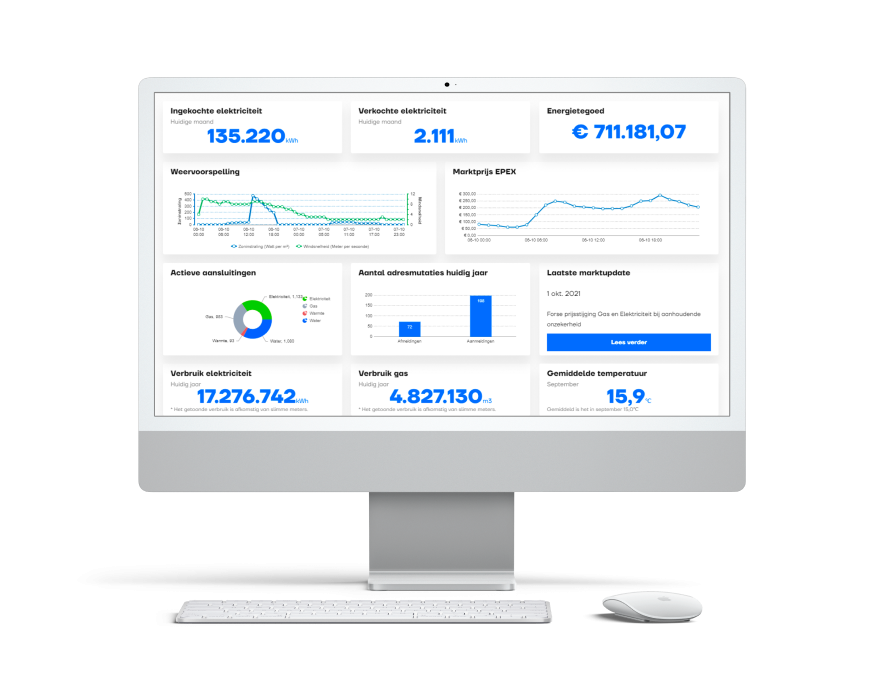
Buy versus Build
To proactively steer within the energy industry, a multifold of different algorithms is required. Building all algorithms internally will not give the velocity in a scaling organization like Groendus, nor is a wise investment decision as external parties can potentially build better forecasting algorithms. Fortunately, there are many providers of energy forecasts, both on volume and pricing up to specific use cases like trading and asset steering. This creates a business case for every energy forecasting use case: I can buy externally against a certain costs, which increase speed of delivery, creates dependency, and guarantees a certain performance and therefore an upside. Or, I can build an algorithm against certain costs, which decrease speed of delivery, creates independency, but potentially outperform (or underperform) the benchmark what is out there in the market.
And that later is important: if there is a large potential to build an algorithm that performs equally good or even better than what’s out there in the market, there is a concrete upside to build it internally (next to increasing a company’s independency). And the key to good algorithms is not the modelling technique and clever thinking, but mostly the data that is being provided. Hence, understanding your internal (and externally available) data quality and quantity and how that can be combined and utilized as an asset in building high-performing algorithms is crucial for the make and buy decision.
Outperforming the existing benchmark
Groendus and Enjins identified energy consumption forecasts as the first algorithm to build in-house, since Groendus has unique data on a low level of granularity about the energy consumption, assets, and energy profile of their customers, which externals do not have. As a result, Groendus had an asset: their data.
The modelling phase started by doing several experimentation iterations, consisting of both extensive feature engineering utilizing the internal (and external) data sources and selecting the time series forecasting algorithm which suited the use case best. Already, the first iterations of the algorithm based on internal data sources, performed equally well compared to the currently purchased benchmark delivered by an external provider. Moreover, building the algorithm in-house enables Groendus to steer on what they want to optimize for: overall model accuracy or reducing total energy costs.
By working on a specific algorithm, the team bumped into many data challenges. Part of every AI use case is to iteratively improve the data, whilst keep on moving and adding the most values as possible within the current boundaries. Hence, the team first focused on a scoped set of clients. From there, proof was built and shared with the organization that created enthusiasm and momentum.
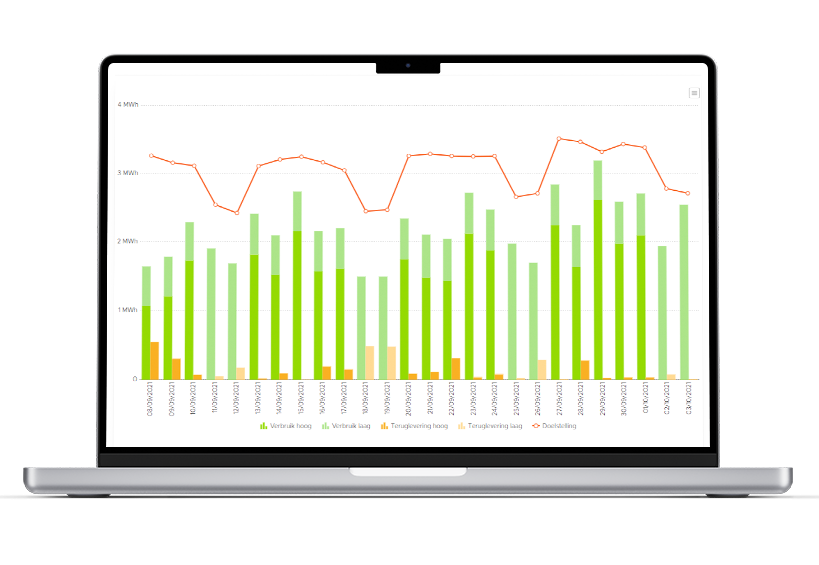
Build an MLOps platform before deploying ML models
Given the investments needed in building a data & AI infrastructure and the challenges to adopt AI within businesses, typically, the first use case will not earn back the investments made. However, spending time on your ML ops platform from the beginning will pay back already in the short-term.
Together with the Groendus team, we set a high standard on how to train, deploy, and monitor the first use case in a standardized way. This is driven by multiple reasons. First, when deploying an algorithm on an infrastructure that is not robust, the team of Groendus had to spend time on maintenance work to get the model running again, instead of spending time on development of new models and improvements on existing models. Second, by standardizing the way to train, deploy, and monitor AI models, multiple people can work on multiple use cases in parallel. And it reduces the dependency to this one data scientist that knows all the ins and outs. Lastly, it speeds up delivery as components are reusable.
As a result, after the first use case was trained and deployed through the ML ops infrastructure, the Groendus team could easily and rapidly build new energy forecasting use cases in a similar fashion.
To scale Groendus’ analytics capability, analytics engineering is key
Next to AI use cases, business intelligence and reporting needs are crucial for Groendus too. The data analytics platform plays a central role as it does not only provide the historical data relevant for machine learning, but that very same data is also used for reporting purposes. Before, data analysts made their reports in PowerBI. It worked well for the first dashboards, but at a certain point it did not scale. Therefore, analytics engineering best practices were implemented to streamline and standardize the analyses built and shared in dashboards.
A key step we have taken was to organize the lakehouse analytics structure through the medallion architecture: having data layers from bronze to silver to gold. Each layer defined by clear playing rules on what data it should entail and how data must flow through these layers. Bronze is the raw data without any alterations. Silver is the clean and integrated layer that adheres to the data model defined within Groendus. The gold layer contains all data sets that are shipped to applications such as dashboards and machine learning use cases. By onboarding young and eager business analysts with some prior SQL knowledge, the data analysts turned themselves into analytics engineers. Now being able to build and streamline analytics pipelines throughout the different layers, utilizing existing code and building new pipelines with engineering best practices.
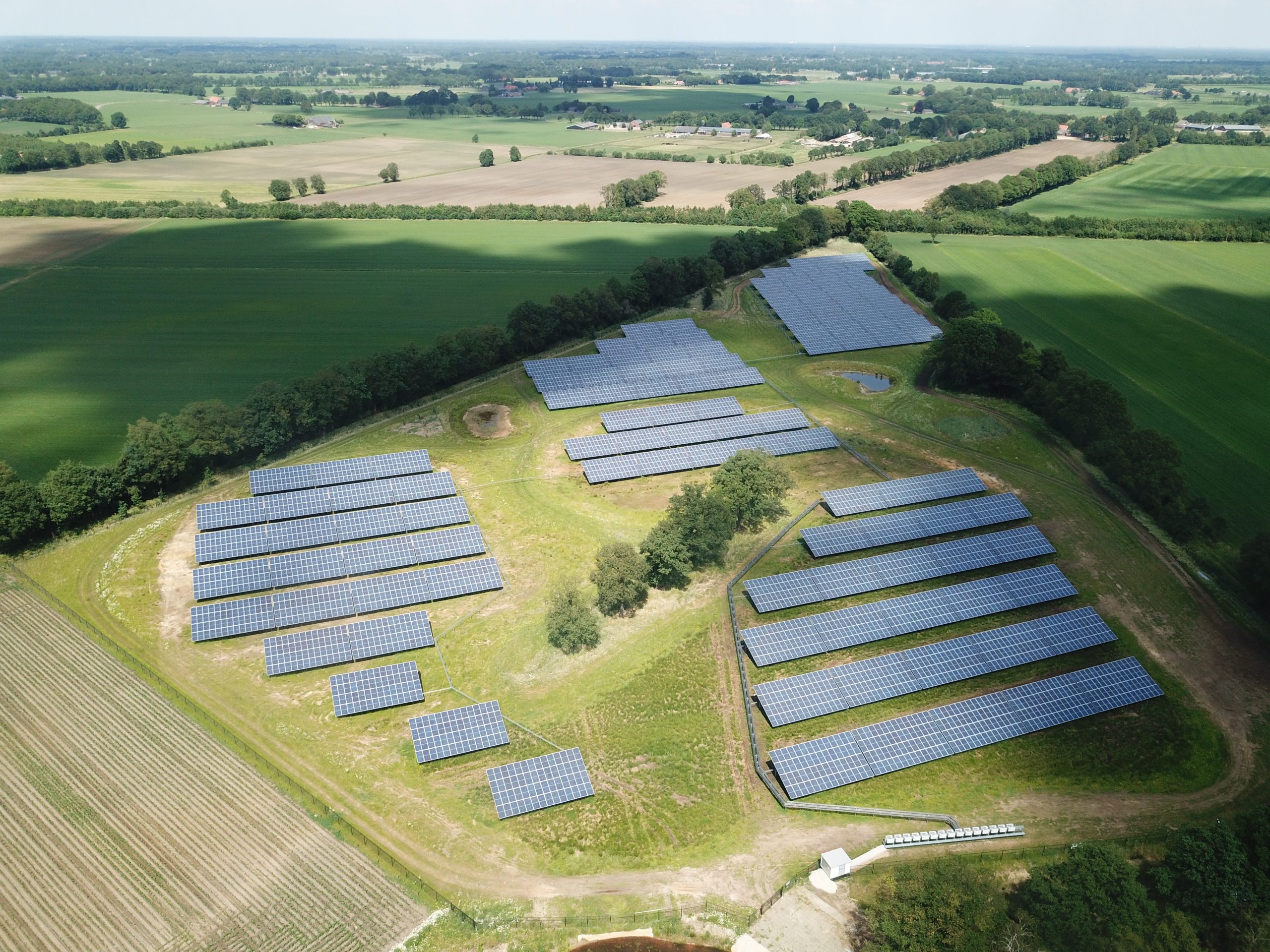
To summarize: Setting up a AI-driven energy forecasting capability requires a solid strategy that enables an organization to make critical make versus buy decisions to spend resources well and to unlock the potential of their internal data that external forecast providers do not have. Moreover, the underlying tech stack plays an important role in scaling both your ML use cases as your analytics use cases. Putting time and effort into your engineering stack always pays off.