Crisp: How we realized a 20% more accurate forecast for 3.000+ products
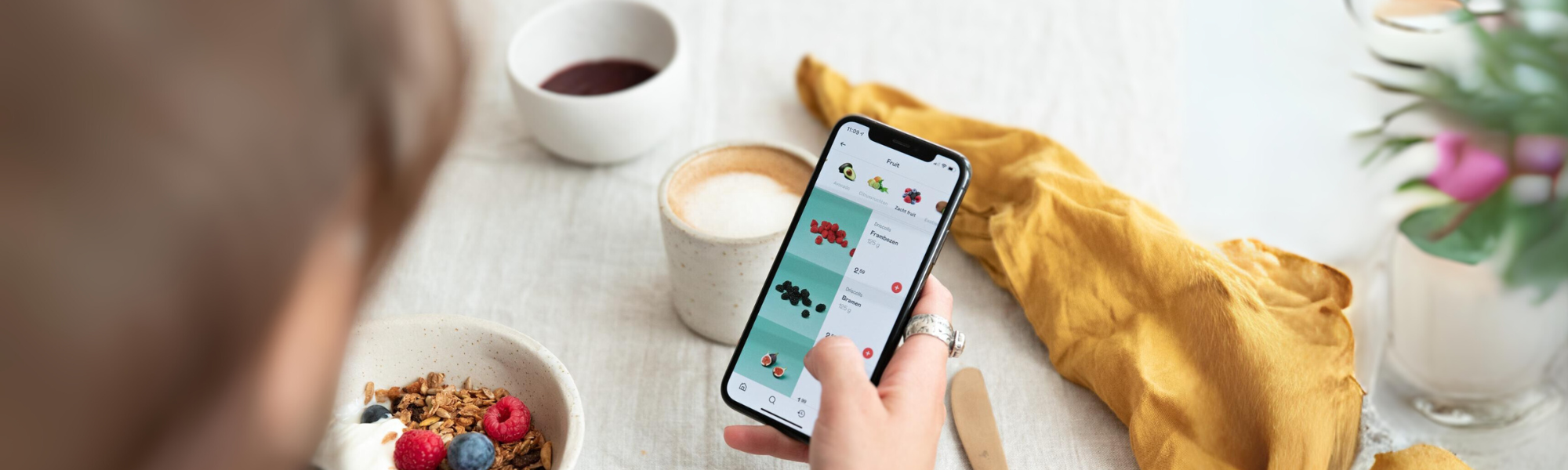
About Crisp
Online supermarket Crisp launched in 2018 and proved to be one of the fastest-growing Dutch startups of the past decade. Customers order products via the app and have them delivered at home. In 2022, the company raised €75M and Crisp is now active in the Netherlands and Belgium. The number of app users is still growing every day.
The need for an accurate forecast
The combination of Crisp’s hypergrowth (meaning a continuously increasing demand side) and the focus on fresh products from 650 farmers and growers (meaning a complex and short-term supply side) makes an accurate product forecast a core company asset for Crisp. Only with such a forecast, supply chains can be optimized for sustainability.
The journey of the last 12 months
In the past 12 months, Enjins and Crisp jointly worked on designing, developing, and implementing a new product forecast into the supply chain process of Crisp. In the following interview, Eric Klaassen (Crisp co-founder) and Erik Heidstra (Crisp head of analytics) look back on these 12 months, including the main challenges and the current impact.
Eric let’s go back to 2018 for a moment. What made you decide to launch an online supermarket like Crisp?
My co-founders and I have a shared love for food. But we noticed the food industry is broken, and good food is hard to get. There are many chains in the food that add limited or no value. We wanted to do things differently, by building a marketplace-like model where consumers could get their daily better food, sourced locally. Since then, we have grown tremendously. I am proud that we have become the #1 online supermarket of the year, for the second time in a row. During the early years of the company, the supply chain experts already developed and used the first forecasting method.
During 2022, the ambition of further maturing this system was growing at Crisp, what were the main drivers here?
On the one hand, scaling in terms of customers and regions means that steering the business and supply chain becomes more complex. Especially since we typically purchase after a customer’s order, but do need forecasts so our suppliers can prepare for sudden changes. An improved forecast helps us to be in control. In control of minimizing waste as well as the risk of being out of stock. Both impact sustainability, profitability, and customer loyalty. On the other side, scaling also means we have more data, so (heavily) investing in forecasting started to make sense. Even for new product introductions, we should now be able to start using data from the past as a first guesstimate of how much one would buy a new product in category x in its first week.
Why did you decide to start collaborating with a company like Enjins on the forecasting topic?
Basically, we had to make two different choices here. The first one was buy versus build. Since we have a unique proposition and adapt to our customers we wanted a forecast model that is just as flexible and can cope with many changes. Since we have built our automated purchase system in-house, it made sense to develop the next forecast system ourselves too, to ensure seamless integration.
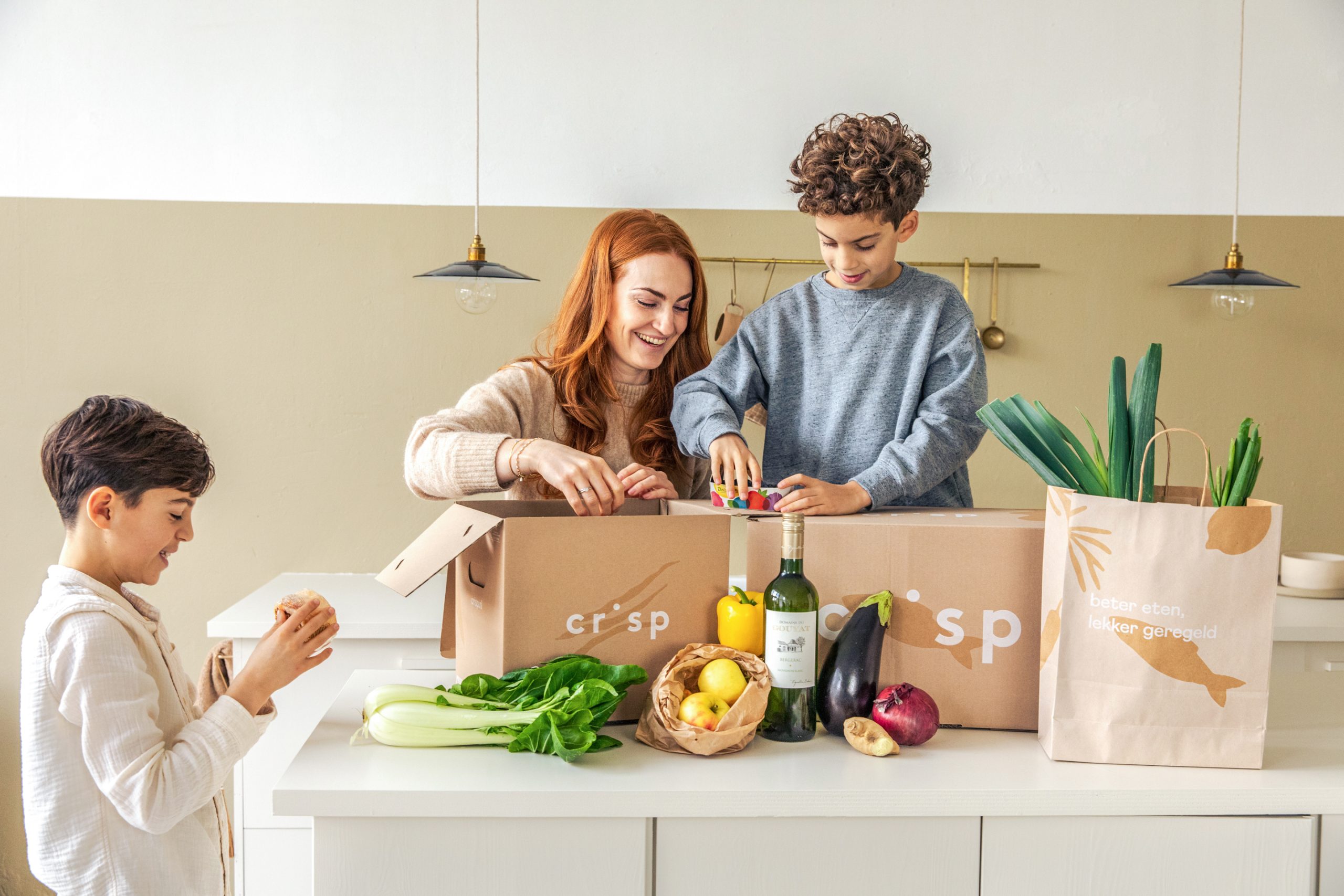
Next, we had to decide whether to rapidly grow an in-house forecasting team or to partner up. To ensure speed we started searching for an external partner.
We liked Enjins for two different reasons; 1) their focus on scale-up customers and understanding of our dynamics and 2) their specific expertise in bringing ML systems and MLOps in production. During the collaboration we grew our in-house team, to ensure smooth transitions of knowledge while developing the forecast.
”I am happy with the results in which we now have a forecast that already improved our main KPI (WAPE = weighted absolute percentage error) with ±20% and a setup in which we can continue to improve the models based on feedback from our experts.
Erik HeidstraHead of Analytics at Crisp
Erik (Head of Analytics). Let’s dive a bit more into the (technical) challenges of forecasting at Crisp. What made the forecasting project challenging?
First, creating an accurate forecast in the context of Crisp is just a challenging job to accomplish. Since we grow so fast in a number of products and customers, we knew that only the last months of data are probably representative, which limits the amount of data you can use to train the models. Secondly, Crisp offers a unique proposition, including recipes, meal kits, and lots of delivery options. Customers display different shopping habits that we need to consider in our forecasting models.
Next to handling these modeling challenges, we had infrastructural and organizational challenges. On the infra side, we had to create not just a model but an ML infrastructure that allowed for model experimentation, deployment, and monitoring in such a way that I could also easily onboard new data scientists in the forecasting project. On the organizational side, it was important to include our supply chain experts during the design and development of the models and system. In the end, the forecast is served to the experts via our Crisp platform (“Kitchen”) and we had to ensure that they understood the predictions and reasoning behind it, such that they could also give valuable feedback. This feedback helped the data scientists to further improve the models.
Let’s zoom-in a bit further into these 3 challenges (forecasting, infrastructure, organisational). Regarding modelling, how did you technically handle all the dynamics of Crisp?
On the modelling side, Enjins really brought in their expertise on how to tackle the forecasting challenge. They took the general forecasting challenges (i.e., dealing with promotion effects and seasonal influences) and the more Crisp-specific ones (i.e., product introductions and fast customer growth) and created a forecasting design that took these into account.
Rather than just choosing the right algorithm we divided the forecasting challenge in different chunks. A four-step approach was used to ensure the different dynamics could be handled.
Customer order forecasting: a model which forecasts the total number of customer orders for a given daypart (morning, afternoon, evening), capturing the company growth trends and general seasonality.
Product forecast: average products per order delivered per warehouse per daypart (also known as shopping cart composition).
Delivery time mapping: the distribution of orders over the possible time slots within a week.
Assortment correction: optimization algorithm which corrects the shopping cart composition and handles interaction effects between products (e.g., when introducing a new red fruit it influences the demand for other available red fruits).
This allowed us to solve these challenges one by one, with many iterations per chunk. In 2022 a first version of the customer order forecast was productionized and our experts started using this. In 2023 we continued with productionising the product forecast and the delivery time mapping. The fourth step is the next challenge that our inhouse team will be working on.
Can you share something about the tech stack used?
Enjins assessed our general (data) infrastructure and created a blueprint of the more ML-specific infrastructure needed going forward to produce the forecasting use case. Altogether, the setup included BigQuery as a data warehouse, DBT for data transformation, Airflow as an orchestrator, and ClearML for model experiments. For ML deployment and monitoring, we jointly developed a fully customized flow tailored to our forecasting process, including a monitoring front-end that integrates into our main Crisp application and can therefore be used by the supply chain experts in their regular working process.
”On the modeling side, Enjins really brought in their expertise on how to tackle the forecasting challenge. They took the general forecasting challenges and the more Crisp-specific ones and created a forecasting design that took these into account.
Eric KlaassenCo-founder at Crisp
Were there any surprises in terms of features that worked way better or worse than you expected?
Well, overall feature engineering proved to be far more important compared to model changes or hyperparameter tuning. One feature that turned out to work well was the ‘giveaway’ feature, which provided information on a specific campaign type that we have at Crisp. Weather also proved to have a significant impact. As you would expect, ice cream is definitely ordered more during sunny weekends :).
How did the buyers experience the project?
They were heavily involved from the very beginning, and we enabled the supply chain managers to overwrite forecasts if needed. Especially in unordinary times or events such as Christmas or a new promotion that has not been done before, we need input from our specialists since the model did not observe these situations before and hence won’t perform well yet in these cases.
Wrapping up, what do you see as the greatest accomplishment of the whole project/collab?
Considering all the dynamics and challenges we had to face, I am happy with the results in which we now have a forecast that already improved our main KPI (WAPE = weighted absolute percentage error) with ±20% and a setup in which we can continue to improve the models based on feedback of our experts. Moreover, my data team now owns all the knowledge since we worked together so closely and we feel comfortable further extending this system going forward.
What’s the main focus for Crisp in the coming 3 years, and how will this impact forecasting?
The food system as we know it is broken and our mission is to fix it. We are becoming the place for consumers for all needs around better food – whether that is groceries, a meal kit, or a ready-made meal. To do so, we continue to focus on sustainable growth – attract customers and wow them with our top service. For forecasting this means more SKUs, more customers, more propositions, and more data. We are continuously working on an even better forecasting model, reducing waste and making Crisp even more efficient.
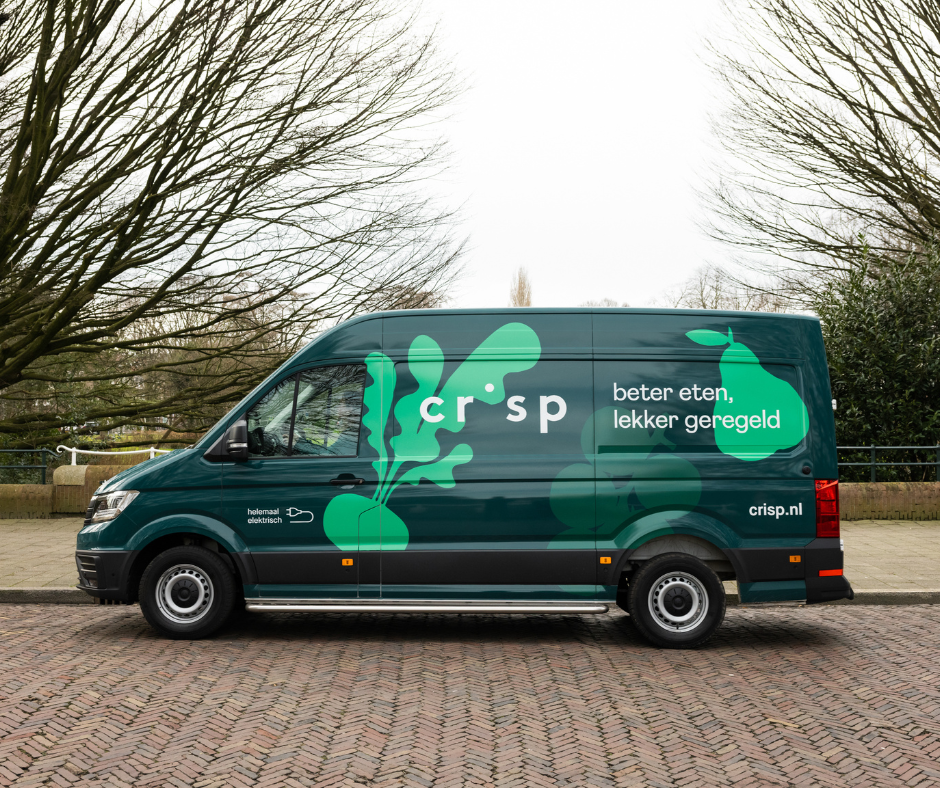